AI and Machine Learning: Harnessing the Power of Automation
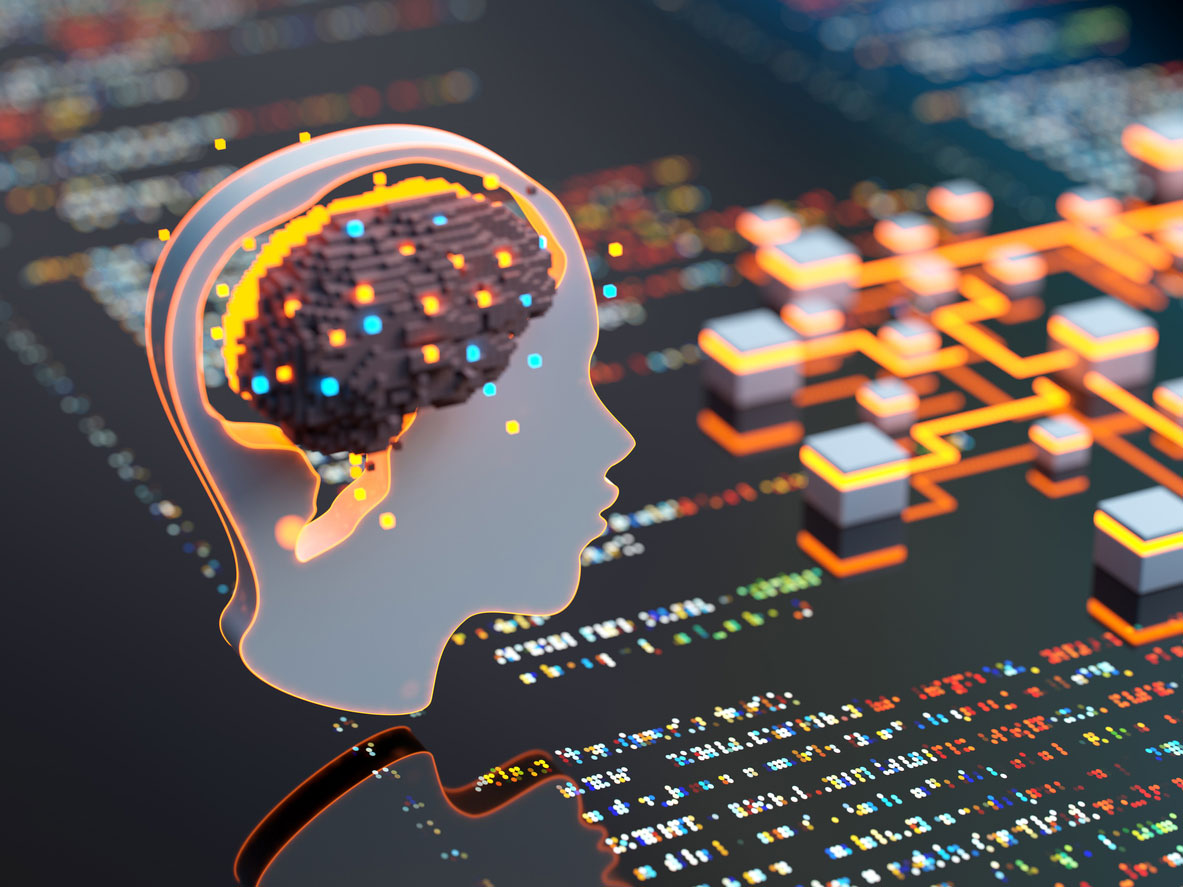

AI and machine learning are transforming businesses in remarkable ways, enabling them to analyze data, automate processes, and make more accurate decisions. The terms artificial intelligence (AI) and machine learning (ML) bring to mind box office cinema tales about elite robot teams or highly dangerous networks that take over the world, replace humans in the workplace, or otherwise disrupt human life as we know it. In reality, AI and ML tools and solutions are created and maintained by humans to complete tasks we can't accomplish or repetitive manual tasks that take too much time.
Artificial intelligence and machine learning both have an important role in industries that range from construction and manufacturing to healthcare. They also have an important place in the evolution of cybersecurity tools used to detect and respond to advanced cyberthreats facing all modern enterprises.
This article covers the basics of AI and machine learning, as well as how businesses are using them to their advantage in cybersecurity.
What is AI & Machine Learning?
The terms artificial intelligence and machine learning are often used interchangeably to describe any application or platform that utilizes automated digital actions. However, AI and ML are two different capabilities that are often used in tandem to create modern solutions for business.
Artificial intelligence is the ability of a machine to imitate intelligent human behavior. It describes the capability of a computer system to complete functions like problem-solving, learning, and planning. The tools accomplish this by analyzing data and identifying patterns within the data provided. Since computer systems don't have the capability to actually reason like humans, they use algorithms to simulate the reasoning that people use when they learn new information to make decisions.
Machine learning is a category of AI that uses data to continue learning without being specifically programmed. Instead of creating complex programming that tells a computer how to perform certain tasks, a software developer can create algorithms that allow the computer to use data to continually improve upon the desired process. Results are improved by using more data to create the program and the introduction of new data to correct mistakes. In many cases, AI is achieved through the use of ML.
Machine learning and AI are the technology behind chatbots, predictive text, language translation apps, facial recognition, virtual assistants, and Netflix or social media suggestions. They're advancing businesses in nearly every industry by streamlining processes and helping teams work smarter. Since ML is used to rapidly consume and categorize large amounts of data, tools that utilize AI can perform tasks that are impossible for humans.
Examples of AI and Machine Learning in use in Cybersecurity
As the evolution of cybercrime continues at a rapid pace, AI and ML have become essential components of effective cybersecurity. Cyber attackers exploit vulnerabilities presented by remote work, cloud deployment, and business digital transformation. These criminals use AI and Ransomware as a Service to gain access to business networks and launch attacks for financial gain or other reasons. To detect these advanced threats and attacks businesses utilize modern sophisticated tools that utilize AI and ML to improve their cybersecurity posture. In fact, AI in the cybersecurity market is expected to grow to reach over $62 billion by 2029. AI and ML are used in cybersecurity to effectively complete these essential tasks.
- Threat and attack detection: SIEM software uses a combination of AI and ML to collect and analyze data and understand the difference between typical network behavior and anomalies that represent a potential threat. The software can be optimized to trigger automated alerts and even take immediate response actions to stop an attack in progress.
- Reduction of alert fatigue: Without the ability to detect patterns in behavior, cybersecurity software can overwhelm analysts with false alerts. Machine learning can create categories for low-risk threats and higher-risk threats. The software can then accurately respond to low-level threats and add context to alerts regarding high-level concerns.
- Identification of zero-day exploits: Central threat intelligence (CTI) uses crowdsourced information from users and clients on a cybersecurity provider's platform, as well as outside sources, to automatically update organizations with proactive protection. By utilizing information from multiple sources, the software can automatically update protection before an organization is affected by a new threat.
- Attack and alert response: While SIEM software is used to detect threats, SOAR (security orchestrated automated response) is triggered to automatically take action when SIEM alerts occur. SOAR uses AI to take appropriate response actions to quarantine threats or shut down systems under attack.
- Elimination of manual tasks: Cybersecurity tools that use AI and ML can rapidly consume large amounts of data produced by a business network. With specific algorithms in place, the tools recognize normal behavior and send prioritized alerts based on the level of risk. As a result, false alerts are decreased. Instead of spending time manually searching data logs and following false alerts, analysts can focus on high-value tasks.
Benefits of AI & Machine Learning in Cybersecurity
In the same way AI and ML streamline processes and allow teams to work harder in various business environments, they increase efficiency and ease difficult processes in cybersecurity. More importantly, the use of AI and automation in cybersecurity increase accuracy in threat detection and response. IBM's 2022 Cost of a Data Breach report listed AI and automation as the cybersecurity solutions that offer the biggest savings, allowing companies to contain a breach 28 days faster and saving 3.05 million in costs.
Automation of Repetitive Tasks
A business network logs thousands of user and entity actions each day. It would be impossible for data analysts to examine every single log entry and identify all of the potential threats a company faces. AI can ingest large chunks of data, use machine learning to recognize patterns on a network and cluster them, and automatically send alerts for potentially malicious behavior. Since ML algorithms keep learning and adjusting with additional data, detection accuracy continues to improve, reducing false positives. Each of these actions reduces the strain on cybersecurity professionals, freeing them up to focus on high-value tasks like improving network protection and threat investigation. This is especially important as the global cybersecurity workforce gap has increased by 26.2% compared to 2021 with 3.4 million more workers needed to secure assets effectively.
Improved Efficiency & Productivity
When your network is compromised, time is your most important commodity. The longer an attacker has access to your system, the more damage can be done. Automated systems analyze data in real-time, detect threats based on known threat patterns and user behavior, and trigger response actions. In 2022, it took an average of 277 days (about 9 months) to identify and contain a breach. By using cybersecurity tools that quickly detect and respond to threats, you can reduce the time attackers spend in your network searching for sensitive data or working to launch a damaging attack.
Increased Accuracy & Faster Decision Making
False positives are mislabeled security alerts indicating threats where there actually are none. They occur for various reasons including the failure to connect patterns that indicate the same action. False positives are a concern because they increase irrelevant noise in an environment that's already overloaded with information, leading to alert fatigue. Machine learning continually adapts to the network environment to identify attack patterns, attach context to relevant alerts, and trigger automated response actions. As a result, data analysts are armed with accurate information about the type of threat or attack, making them better equipped to provide the right response.
Cost Savings
AI and ML reduce the effort required to detect and respond to cyber threats, resulting in cost reduction. Automated tools also reduce the strain on your cybersecurity team. The cost of a fully staffed SOC located on the premises of your business is out of reach for many businesses. The use of AI and ML tools as part of a comprehensive cybersecurity solution provided as a service can cut your costs significantly in comparison. Additionally, the use of such effective solutions cuts costs of damages and ransom related to successful attacks.
Enhanced Immunity Against Future Threats
ML algorithms are designed to continually consume data within an environment to keep learning. With the ability to detect new and changing patterns that indicate a threat, these tools can alert professionals to suspicious behaviors before attackers reach their goals. UEBA capabilities make AI tools superior at recognizing behaviors outside the norm in your business environment, an ability that is crucial to identify social engineering or credential theft attacks.
Detection is not the only method of protection provided by AI and ML in cybersecurity. Central threat intelligence utilizes threat information from a variety of sources to validate threats and automatically update your network with proactive protection against evolving threats. When AI is applied to perimeter tools like firewalls and cloud protection, automated services like vulnerability scans and updates provide protection against new vulnerabilities and evolving threats to add a new layer of protection to your network without human intervention.
The Future of AI and Machine Learning
The global AI space is expected to grow to $20 billion by 2025. AI applications are sued daily in HR, accounting, healthcare, etc., to manage, store, and transfer large amounts of customer data. AI-driven tools help medical professionals diagnose and treat patients more quickly and accurately. AI technology is used in transportation and freight to track mobile assets.
As AI and ML grow across practically all industries, it's difficult to comprehend what the future might hold for the technology. Some predictions suggest that AI will write best-selling books by 2049, and perform surgeries by 2053. Advances from Tesla alone suggest that autonomous cars will eventually be commonplace. Telehealth advancements in the healthcare field are expected to advance, allowing patients to receive a significant amount of care from home.
In more general terms, AI and ML will likely be used across all industries to integrate and streamline processes in ways that haven't been anticipated in the past. Large network environments will likely depend on a series of AI and ML enabled tools that wirelessly communicate with one another to seamlessly conduct daily functions.
While AI and ML are already vital for the protection of multi-cloud organizational environments, tools with these features are likely to evolve further in cybersecurity. As businesses depend on more extensive networks with remote devices and other endpoints, ML will be used to identify and profile devices on a network. ML continually becomes more advanced in language normalization and the ability to communicate with humans. As such, there is increased potential for AI tools to provide targeted and accurate security hygiene suggestions for businesses and response actions for cybersecurity professionals. Perhaps most importantly, ML has the potential to increase integration capabilities which can eliminate vulnerabilities created by tool sprawl and multiple dashboards.
Artificial intelligence and machine learning enable tools to quickly add context to vast databases and work around the clock. Furthermore, the technology allows machines to consistently learn and improve with the introduction of new data. When these capabilities are implemented in cybersecurity, organizations gain the benefits of 24/7/365 security that is capable of recognizing zero-day attacks, stopping threats in progress, and even recognizing credential theft through anomalies in behavior. Currently, AI and ML are an essential part of maintaining protection against cyberattacks that are constantly evolving in sophistication. In the future, AI is likely to take center stage in all cybersecurity offerings.
Machine learning and artificial intelligence alongside human intuition and education are a powerful combination. Even as concerns are rising about the increased capabilities of cyber criminals, cybersecurity professionals continue to develop tools and methods that keep business networks safe from attack. As businesses evolve along with technology, the demand for effective cybersecurity will grow continually. The use of AI and ML in cybersecurity will continue to help alleviate the difficulties related to the growing talent shortage in the industry.
Cybercriminals use all of the tools at their disposal, including AI and as-a-service attacks. Your business should be using modern tools to combat these attacks. BitLyft Air® is an automated threat detection and remediation platform that combines multiple security offerings into one single solution. Learn more about how we can provide total protection for your entire business network.
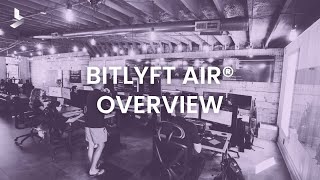